Aira: a state-of-the-art ai-powered tool for retial analysis and disease diagnosis
In the modern age of technology, there are still people who lack access to state-of-the-art medical diagnosis and treatment. AIRA (AI Retina Analyzing and Disease Diagnosis Tool) brings them a step closer to providing reliable and cost-effective ophthalmological care to the masses.
AI and data scientists are now joining hands with health care professionals to develop tools that aid in providing specialized medical care to the masses. There is still a lack of available niche medical services to people globally, particularly in underdeveloped countries. Fortunately, the field of AI and machine- learning has made significant progress in developing applications for diagnosing illnesses, tabling treatment options and monitoring patients. They have also inbuilt databases with valuable information that is supplementary and complementary to physicians or doctors.
AIRA, by Distributed Hub, was developed by a team of leading developers and ophthalmologists. It uses the latest methods in AI and machine learning for the diagnosis of retinal diseases. AIRA has an extensive database of various symptoms and pathological cases used for pinpointing various conditions related to retinal diseases. The tool can also be used to monitor retinal disease and its progress prediction.
AIRA was developed by integrating advanced techniques in biomedical image processing, computer vision, deep learning and AI. Each area presents its own challenges despite major advancements in their respective fields.
Challenges in Biomedical Image Processing
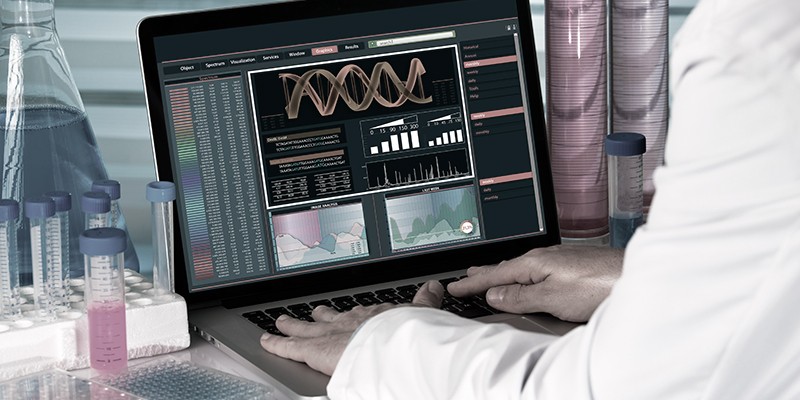
The importance of biomedical image processing cannot be denied considering the need for automated systems for health care. Biomedical image processing systems can be distinguished apart from typical computer vision frameworks for several reasons. Following is a list of key challenges faced by scientists working on processing medical images and extracting meaningful information from them:
Task Complexity
Typical computer vision applications dealing with image classification require the prediction of a single label corresponding to an input image. For biomedical images, the task is more complex as localized areas containing diseases or abnormalities have to be pinpointed, their boundaries marked accurately and proper segmentation of input images required.
Lack of Accurately Labelled Universal datasets
Accurate labelling of medical images requires skilled and trained professionals. This is unlike the general datasets like ImageNet, widely available and used by the computer vision community. Images constituting ImageNet can be labelled manually by a layperson making it easy to add more high quality labelled data points to the existing dataset. However, when it comes to biomedical images, there is very little accurately labelled data available, making it difficult to train deep learning models. For different specialized fields of medicine, there is a limited choice of professionals who can accurately label them.
Diversity of Images
Biomedical images can be derived from a variety of sources like ultrasound, CT scan, MRI scan etc. Each mode of image acquisition and the information it conveys is different. Images derived from different sources may require an extra layer of preprocessing which would be specialized to its source.
Deep Learning For Biomedical Image Analysis and Specialized Medicine
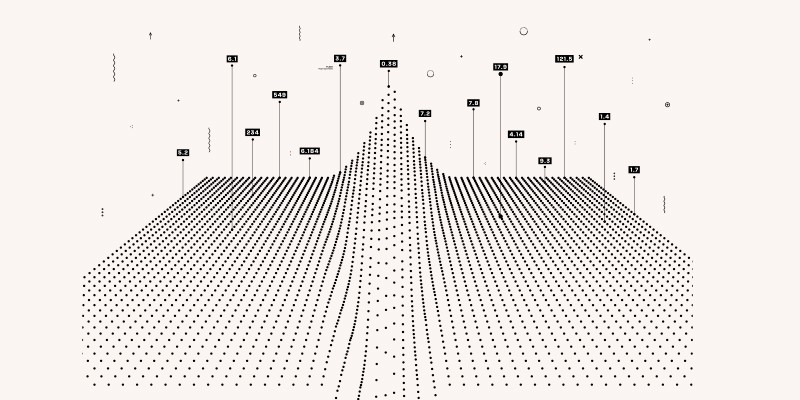
Deep learning architectures are the focus of attention these days due to their proven ability to solve a variety of complex tasks. Originating from traditional neural networks, deep learning architectures coupled with present-day high computational power and a large number of neuron processing units arranged in several layers, have demonstrated superior performance over humans for many tasks.
CNNs (convolution neural networks) have proven their worth for providing a high degree of accuracy on image classification problems. Generally for CNNs, the input is an image and the output is a label.
However, for biomedical images, diseases or abnormalities present in different regions of the image also have to be identified. A research group at the University of Freiburg in Germany developed UNET, a specialized version of CNNs, specifically designed for this purpose.
U-NET labels each pixel of an image and hence the size of the output is the same as the size of the input. This structure helps localize the regions exhibiting an anomaly or deformity. A noteworthy feature of U-Net is that it requires a small number of training data points for accurately and efficiently segmenting and classifying images. Given this feature, U-Net has gained popularity in solving problems related to specialized medicine and biomedical imaging.
Combating Retinal Diseases Using Technology
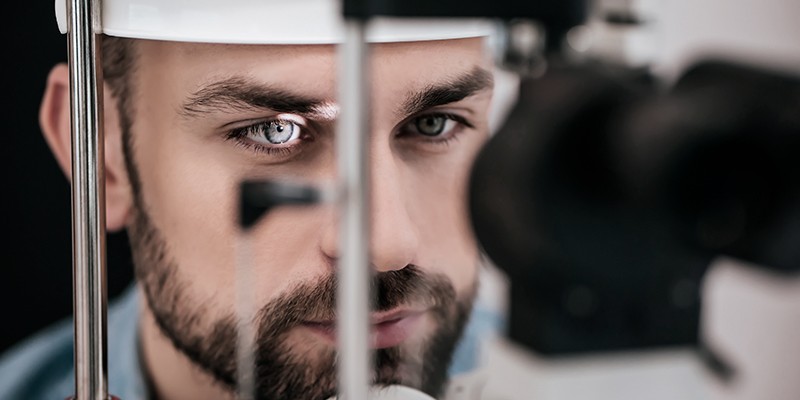
One area of specialized medicine is retinal diseases. The retina is an important part of the eye, located in the posterior segment inside the eye. It consists of thin neural tissues, which are sensitive to light and are used to communicate visual information to the brain via the optic nerve. Any retinal damage or disease requires immediate diagnosis and treatment. Delayed or untreated cases can result in permanent loss of visual acuity.
An important part of diagnosing retinal diseases is by taking special images called fundus images. Fundus is the interior surface of the eye, constituting the retina, optic disc and other parts of the interior surface. Fundus cameras are high precision tools consisting of a microscope and a camera. Digital fundus images help optometrists or ophthalmologists diagnose various eye or retinal disorders.
Diagnosis of retinal diseases requires specialized personnel in optometric and ophthalmology. The assistance of a highly-skilled, experienced doctor may be needed to read and interpret the fundus/retinal images. This is where deep learning-powered machine learning algorithms can play a part, i.e., recognize and pinpoint the presence of disease in retinal images and suggest various treatment options.
The lack of a universal knowledge base of different areas of specialized medicine makes it hard for young or inexperienced doctors to make accurate diagnoses. Same is true for many retinal symptoms and diseases. AI and data science can help specialists to collaborate and add to this knowledge base.
AIRA: A Solution by Distributed Hub For Diagnosing Retinal Diseases
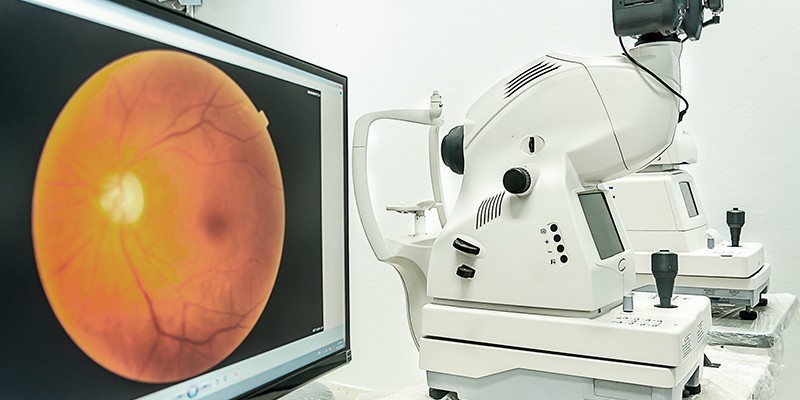
Leading AI and machine learning developers at Distributed Hub teamed up with ophthalmologists to provide a high-tech solution for automated retinal symptoms identification and diagnosis of various diseases. The key features of AIRA that distinguish it from other similar tools are:
- Identification of various symptoms present in the anatomical structure of the retina.
- Accurate and reliable diagnosis of retinal diseases.
- Extensive knowledge base of pathological cases.
Data Collection
The development team at AIRA was provided with a large dataset of retinal images with the image masks for the following symptoms, and morphological and anatomical features:
- Exudates;
- Hemorrhages;
- Microaneurysms;
- Disk;
- Cup;
- Drusen;
- Veins;
- Arteries.
To build a comprehensive dataset, the images were collected from different sources by using different cameras with varying qualities. The image quality varied depending upon the camera and the opacity of the eye structure. Some images also had minor visual artifacts adding to the diversity of data.
Data Preprocessing
Many machine learning algorithms require data to be in a consistent and uniform format. Same is true for neural networks that require a fixed size for all inputs. All data images were hence converted to RGB format. They were normalized and resized if needed.
Data Generation
When training deep learning networks, it is common practice to increase the size of available data by synthetically generating new data points. The dataset for AIRA was augmented by generating new images by employing the following techniques:
- Affine transformations such as rotations, shift, skew etc.
- Change of color and quality by applying various filters like blurring, contrasting, hue, saturation, etc.
Training via U-Net
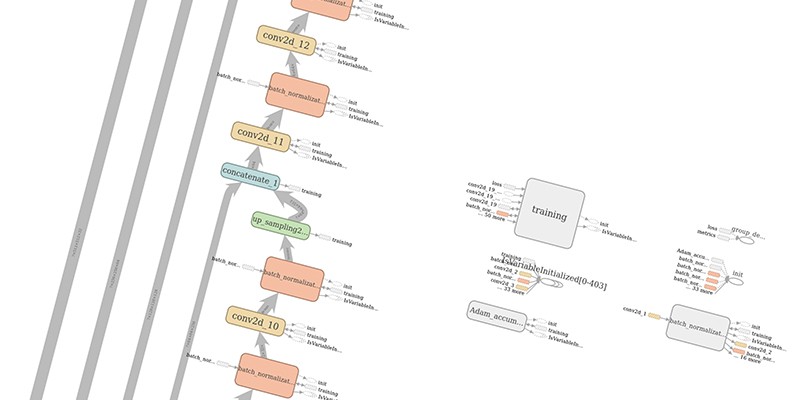
U-Net, derived from CNNs, has several contracting layers followed by expansion layers. Its architecture gives it a “U” like appearance and ensures feature maps learnt in the contracting layers are directly passed on to expansion layers used for reconstructing output segmented image maps.
Distributed Hub’s team developed a highly specialized deep learning model based on U-Net after several trials and extensive experimentation. The technical features in the final network architecture feature:
- Activation function: ReLu;
- Using layers of batch normalization: + Method of increasing the dimension: Upsampling;
- Count of filters in the mid feature vector: 512;
- The dimension of the input/output layer: 512×512;
- Optimizer: Adam Accumulate;
- Missing data: Extrapolation by mirroring.
Implementation via the above parameters resulted in precise and accurate segmentation and prediction while using fewer training images. Integrating AIRA in a fundus camera would enable novice and inexperienced doctors to accurately and reliably diagnose retinal diseases. Skilled doctors can use AIRA’s platform to train a network using their own specialized images adding to the knowledge base.
Example Results
The advantage of employing U-NET is that each image is labelled pixel by pixel to identify various structures or anomalies present in the retina. Two broad categories identified by AIRA are anatomical structure or a symptom. Examples of the two different tasks are illustrated below. In each figure, the input image is shown on the left and the identified anatomy or symptom is shown in color on a gray-scaled background.
Identification of Anatomical Structure
Shown below are examples of arteries and veins identified by AIRA:
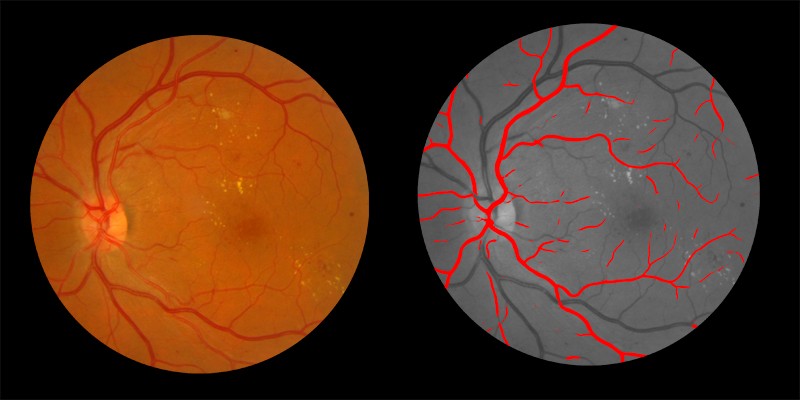
Arteries Identified by AIRA shown in red on the right
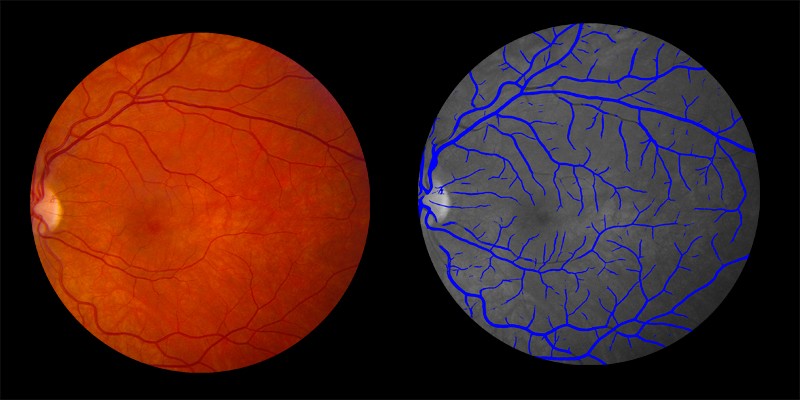
Veins identified by AIRA shown in blue in the right image
Symptoms Identification
Two examples of symptoms pinpointed by AIRA are shown below:
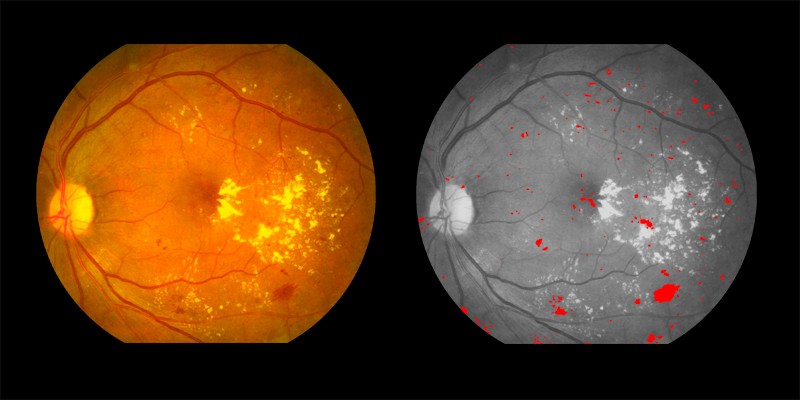
Hemorrhages shown in red in the right image
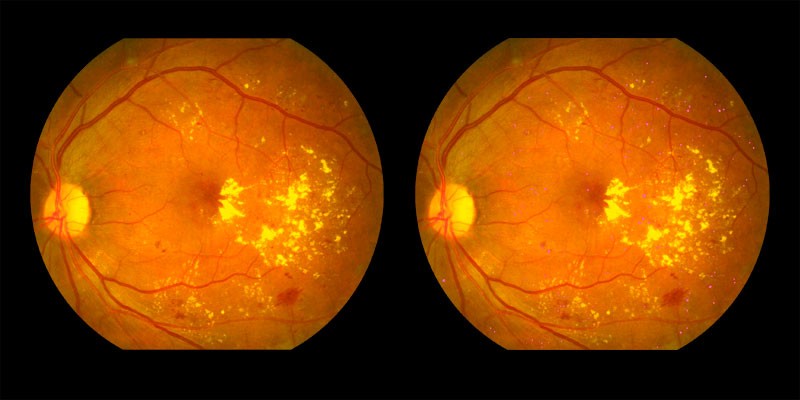
Signs of microaneurysms shown by the pink dots
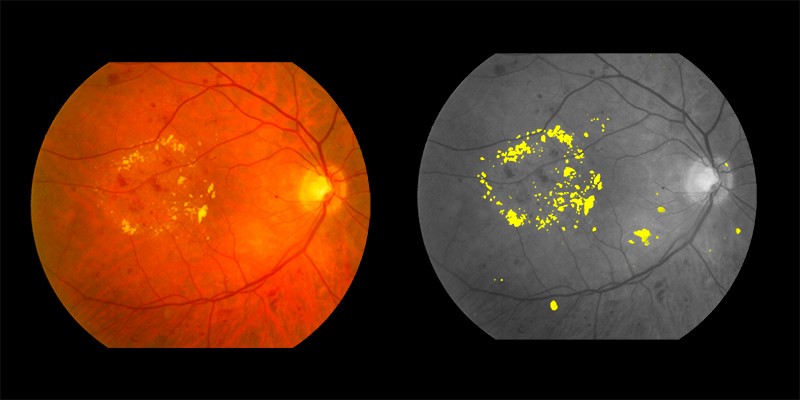
Exudates shown in yellow in the right image
Contribution to a Universal Knowledge Base
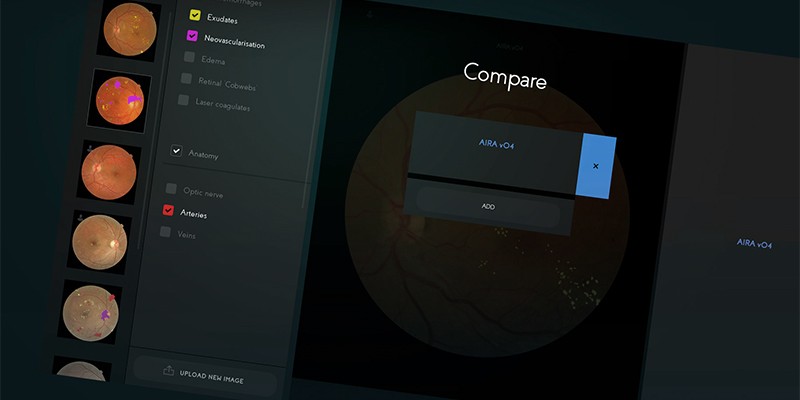
AIRA has been trained on a set of retinal images making it a general platform for retinal disease diagnosis and aid inexperienced doctors making an accurate diagnosis. Ophthalmologists can provide their own training images with masks for additional symptoms and anatomies to further specialize the system for other retinal pathologies. This would enhance the analysis and prediction of the neural network and broaden its domain to cater to a wider set of retinal pathologies.
Large numbers of people around the world do not have access to specialists despite having investigative tools like a fundus camera. AIRA is a valuable tool that can help medical staff make an initial assessment of retinal diseases in patients. AIRA can also help monitor patients as the disease regresses and to define the difference in symptoms as percentages, which humans would find hard to do; and serve as an aid to making referrals to specialized doctors if needed.
Path for the Future
A completely automated framework for specialized medicine still sounds like a futuristic system but technology, data scientists and AI algorithms have progressed enough to make it a realistic aim. AIRA has made headway in the development of platforms that bring cheap and reliable retinal care to the masses. It is the perfect tool that can be used by doctors to make accurate and reliable diagnosis and offer treatment options. The lessons learnt in developing AIRA would help future machine learning scientists to develop similar systems in other fields of specialized medicine.